

This is primarily due to the fact that the RSSI values are unstable because of higher noise in the BLE tag signal, thus producing localization results that may be inaccurate. However, affordable implementations of this method may have difficulties locating BLE tags with the conventional received signal strength intensity (RSSI) triangulation method. Due to the accessibility of BLE tags and detectors, this technology is versatile and can be used in combination with various hardware.
#RADBEACON DOT UPDATE FREQUENCY BLUETOOTH#
On the other hand, Bluetooth Low Energy (BLE) technology provides an alternative method for indoor tracking. Unfortunately, the proprietary hardware required for this system is expensive and requires extensive cabling. Currently, the main-stream commercial method that is used for indoor tracking is radio-frequency identification (RFID) technology. In order to monitor clinical workflow, it is essential to develop an accurate real time location system (RTLS) to track patients and clinical staff. By monitoring biometric and workflow data, a smart clinic consisting of various deep learning models may play a large role in improving the efficiency and safety of a clinic, especially in the setting of a radiation oncology facility where quality assurance and patient safety are a primary concern. Finally, this model is tested by employing an independent testing data set which the network has never encountered. This data set is then used to train a neural network model to perform the desired task. First, a training data set is collected and properly labelled. With proper training, supervised learning techniques such as convolutional neural networks are capable of analyzing large data in order to yield meaningful results.
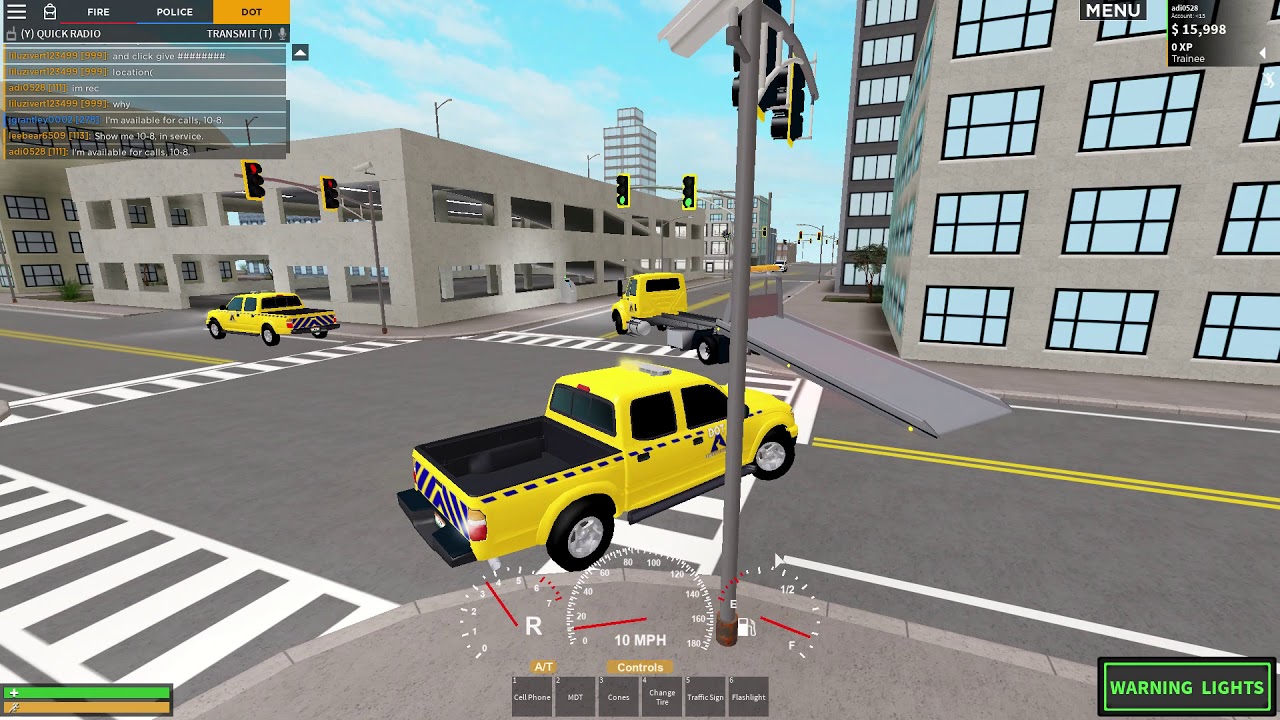
However, it is infeasible for physicians or other medical staff to constantly view these biometrics in real time to detect any abnormalities in a patient’s condition.įortunately, continuous monitoring is possible by utilizing deep learning methods. Furthermore, with the proliferation of wireless technology, a patient’s necessary biochemical information can be transmitted directly to a clinic from almost anywhere in the world. Wearable sensors provide a new avenue for ensuring patient health by providing physicians with tools to monitor several biochemical metrics, including blood pressure, oxygen saturation, heart rate, and several others, outside of the treatment facility. In order to carry on this evolution, technological advancements continue to be integrated and adopted into the clinic. The advent of innovative technologies has fueled the progression of cutting-edge treatment and quality patient care. In recent decades, the field of medicine has evolved at an alarming rate. png format and are in labelled folders, which makes the data easy to read and classify based on directory location.įunding: The authors received no specific funding for this work.Ĭompeting interests: The authors have declared that no competing interests exist. These images comprise the entire data set (more than the minimal data set shown in the paper). This is an open access article distributed under the terms of the Creative Commons Attribution License, which permits unrestricted use, distribution, and reproduction in any medium, provided the original author and source are credited.ĭata Availability: All of the images used for the research are uploaded to the Github repository. Received: DecemAccepted: SeptemPublished: October 11, 2018Ĭopyright: © 2018 Iqbal et al. Queen Mary University of London, UNITED KINGDOM (2018) Accurate real time localization tracking in a clinical environment using Bluetooth Low Energy and deep learning. Future studies will seek to deploy this affordable real time location system in hospitals to improve clinical workflow, efficiency, and patient safety.Ĭitation: Iqbal Z, Luo D, Henry P, Kazemifar S, Rozario T, Yan Y, et al. It outperformed a CNN model (accuracy = 94%), a thresholding model employing majority voting (accuracy = 95%), and a triangulation classifier utilizing majority voting (accuracy = 95%). By utilizing temporal information, a combined CNN+ANN network was capable of correctly identifying the location of the BLE tag with an accuracy of 99.9%. The performance of these networks was compared to relative received signal strength indicator (RSSI) thresholding and triangulation.
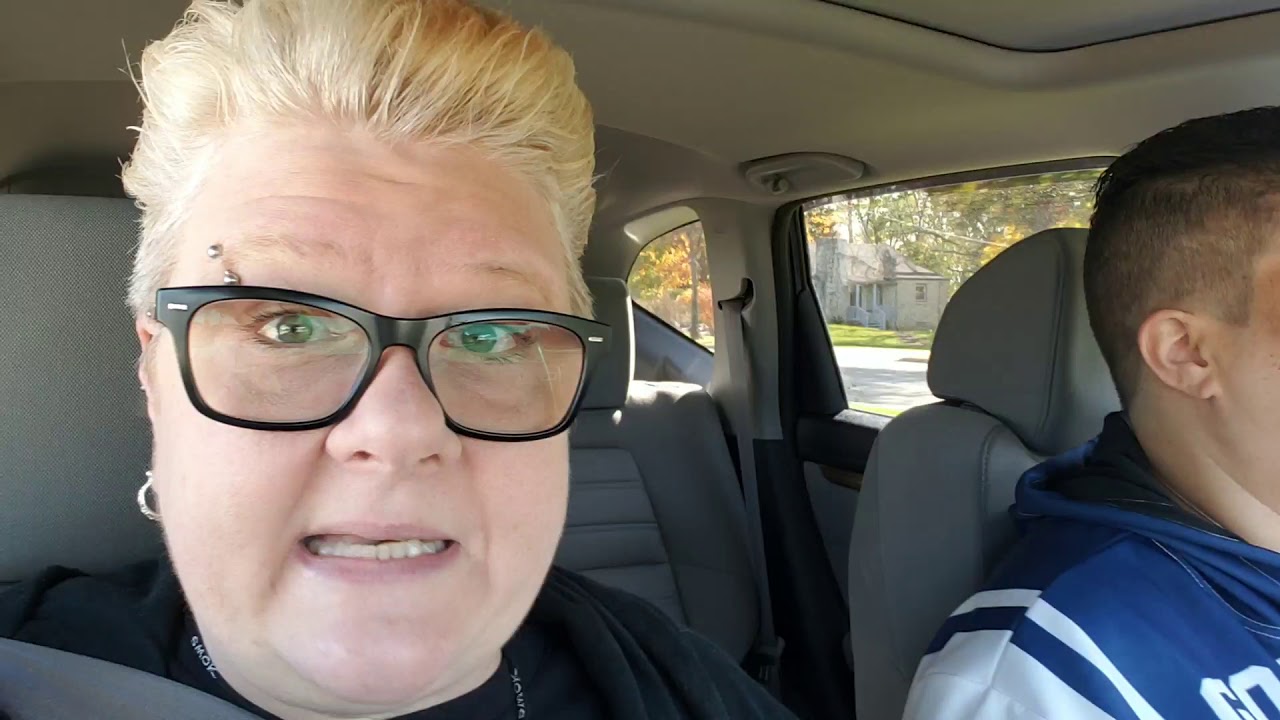
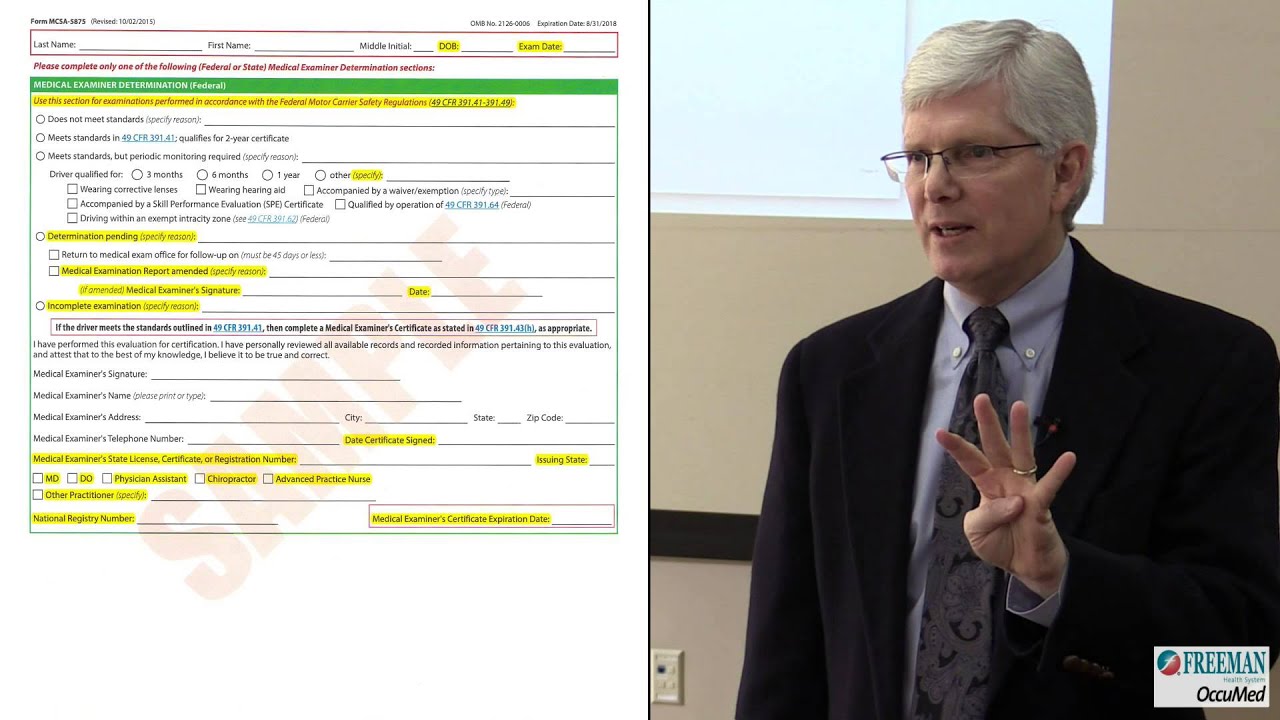
This study focuses on investigating the feasibility of tracking patients and clinical staff wearing Bluetooth Low Energy (BLE) tags in a radiation oncology clinic using artificial neural networks (ANNs) and convolutional neural networks (CNNs). Deep learning has started to revolutionize several different industries, and the applications of these methods in medicine are now becoming more commonplace.
